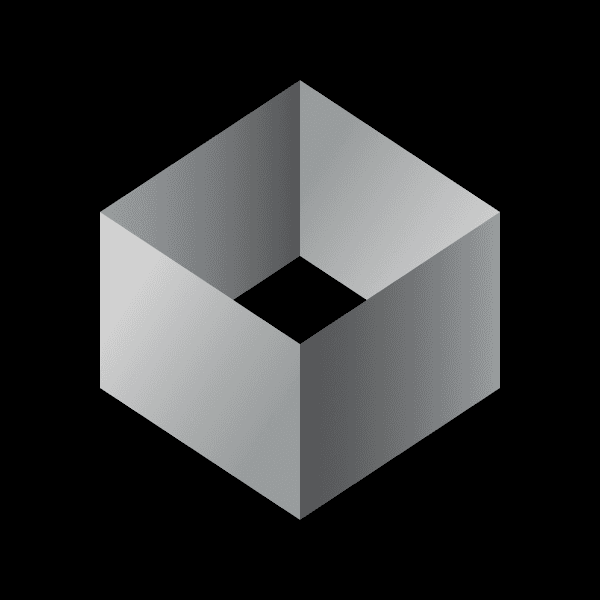
Iterate AI
Sep 20, 2024
If you're looking to create products that truly live with your users, then product analytics is something you should know about. It's all about understanding how people interact with your product and using that knowledge to make smart decisions.
So, imagine you are building a new app, you might think that your users want X, Y and Z features, but seriously, do they need them? Unless you start tracking, you don't know if the users are using that feature.
This is where product analytics comes in.
By collecting the analytics data on how often users are using the app, what features they are using, where they are getting stuck, etc, you can determine what needs improvement.
For example, let's say you notice that a ton of people download your app, but hardly anyone makes it past the first week. That's a red flag that something in your onboarding process isn't clicking.
But if you dig deeper and see that the ones who do stick around are the ones who added at least 10 friends in their first few days, you know where to focus your efforts.
That's exactly what Facebook did in their early days. They realized that getting new users to connect with friends was the key to keeping them engaged long-term.
So they made it a priority to get people to that 10-friend milestone as quickly as possible. This was a turning point for their growth; check out this video to learn more about Facebook growth to 1B users:
Why does product analytics matter?
Product analysis fits perfectly for businesses of all sizes as it provides valuable insights into user behavior, helping businesses make informed decisions for growth and user satisfaction.
Here is why product analytics matter.
Informed Decision-Making: Product analytics allows businesses to base their strategies on actual user data rather than assumptions. And with this better product development, marketing, and customer engagement strategies.
Improving User Experience: By understanding how users interact with a product, businesses can identify pain points and optimize features; this will help in a more satisfying user experience.
Increased Retention and Engagement: Analytics can tell which features keep users coming back. With the help of this, businesses can improve user retention and engagement rates.
Competitive Advantage: Companies that are using product analytics effectively can differentiate themselves from competitors by enhancing or pivoting their offerings to meet user needs.
Case studies of brands that benefited from effective product analytics
We discussed Facebook and their 10 friends approach. Let’s see other brands that have been leveraging product analytics.
1. Spotify
Spotify analyzes data on how users discover, listen to, and engage with music on their platform. By studying listening patterns and preferences, they can create highly personalized playlists and music recommendations for each user.
This makes sure that every Spotify listener gets a unique experience tailored to their tastes.
As a result, users are more engaged with the app, less likely to churn, and more willing to pay for a premium subscription.
Read more here: Spotify Product Analytics Case Study.
2. IKEA
IKEA uses product analytics to optimize both their online and in-store shopping experiences.
On their website, they track how customers navigate product pages, add items to their carts, and complete purchases. This data helps them simplify the online buying process and highlight popular products.
In their physical stores, IKEA uses analytics to determine the best placement for items, ensuring high-traffic areas showcase their most profitable furniture and decor.
By understanding how customers shop, Ikea can guide them to the products they're most likely to buy, leading to higher average order values and sales.
Read more: Ikea Product Analytics Case Study.
3. Supercell
Supercell uses product analytics to track player engagement and spending patterns in their mobile games, Their popular games are Clash of Clans, Clash Royale, Brawl Stars, etc.
By analyzing user data, they identify which features keep players interested and where they lose engagement, allowing them to make informed decisions about game updates and new content.
This data-driven approach has helped Supercell maintain high player retention and profitability in a competitive market.
Read more: Supercell Product Analytics Case Study.
Now let’s move on to the stakeholders in product analytics, and how they make the most out of it.
Key Stakeholders: Who Uses Product Analytics?
A simple breakdown of how different roles within a company can leverage product analytics.
Executives and Leadership: For making strategic decisions about product direction and investments by reviewing key metrics and reviewing the product's health and alignment with business goals.
Product Managers: For tracking user engagement and identifying valuable features to prioritize product enhancements and align strategies with user needs.
Product analysts: for interpreting data collected from users, identifying trends, and providing insights that inform product strategy.
UX Designers: For understanding user interactions, examining user flows, and identifying issues to improve the overall experience and increase user satisfaction.
Sales Teams: For understanding customer behavior, tailoring pitches, and identifying opportunities for upselling based on how potential customers interact with the product.
Marketing Teams: For campaign effectiveness, understanding customer behavior, tailoring strategies, optimizing messaging, and targeting the right audience to drive customer acquisition and retention.
Customer Support Teams: For gaining insights into common user issues, understanding which features users struggle with, and addressing concerns to improve overall satisfaction.
B2B vs B2C Product Analytics Difference
Before discussing the metrics to track, let’s differentiate between product analytics in B2B and B2C.
Difference between B2B and B2C Products.
For B2B Product Analytics
High Complexity, Low Volume: As B2B companies have a smaller number of customers, each account's data is deeply analyzed.
Product teams focus on understanding how various roles (e.g., managers, IT teams, and end-users) interact with the product. A single B2B client might have several users, each requiring different features, this makes the analysis more customized.
Cross-Department Insights: Analytics in B2B products often combine product usage data with CRM data to understand account health and growth potential.
For example, if multiple users from the same company use key features, it signals potential upsell opportunities.
Long-Term Engagement Focus: As B2B sales cycles are long, product analytics isn't just about short-term conversions. The key is to understand long-term engagement and how clients use the product over time to drive renewals and upgrades.
For B2C Product Analytics
High Volume, Simpler Data: B2C analytics must process large volumes of data, often automating insights through dashboards and machine learning.
Clickstream data, purchase history, and app behavior give product teams a snapshot of customer needs.
Instant Feedback, Quick Adjustments: B2C businesses rely on immediate feedback loops to optimize their products. For example, a streaming service might track how introducing a new "Skip Intro" feature affects user retention and increases watch time.
Behavioral Patterns: In B2C, customer behavior can be analyzed en masse to find common patterns. For example, identifying a high drop-off rate on the checkout page can lead to UI/UX improvements to boost conversions.
Product Analytics vs. Other Analytics
Unique Features of Product Analytics
Product analytics is a specific (as the name says) branch of data analytics that focuses specifically on understanding user behavior and product performance.
User-centric insights
→ Understand user preferences and behaviors
→ Optimize user experience and product features
→ Monitor user actions (clicks, usage)
→ Identify popular or underutilized features.
Behavioral Segmentation
→ Segment users by interactions
→ Tailor marketing and improve engagement
Real-Time Feedback
→ Get immediate insights into user behavior
→ Quickly respond to trends and issues
Longitudinal Analysis (it is understanding the same variable over time)
→ Track changes over time.
→ Evaluate the impact of updates on engagement.
Integration with Business Goals
→ Align product decisions with business metrics (revenue, retention).
How to Choose the Right Product Analytics Solution
A simple Q&A for choosing the best product analytics solution.
Q: What should I consider regarding team size?
Small Teams: Look for tools with user-friendly interfaces and easy setup.
Large Teams: Opt for solutions with robust collaboration features and scalability.
Q: How does product complexity affect my choice?
Simple Products: Basic tools with essential features may be sufficient.
Complex Products: Advanced tools with detailed tracking and segmentation are beneficial.
Q: How important is budget in selecting a tool?
Assess the cost against the features offered.
Consider free trials or freemium models to evaluate before committing.
Q: Why are integration capabilities important?
Ensure the tool integrates smoothly with your existing tech stack (e.g., CRM, marketing platforms).
Q: Should customization and flexibility be a priority?
Yes, choose a tool that allows customization to fit your specific analytics needs.
Q: Is real-time analytics necessary?
For immediate insights, select tools that offer real-time data processing.
Q: How do support and community play a role?
Evaluate the level of customer support and the presence of a user community for troubleshooting and advice.
Now let’s look at some product analytics solutions.
4 Best Product Analytics Tools to Check Out
PostHog
PostHog is an open-source product analytics platform that allows you to track user behavior, run experiments, and manage feature flags all in one place.
Self-hosted option for complete data control
Built-in feature flagging and A/B testing
Session recording and heatmaps for user behavior insights
Also read: Posthog Autocapture feature and alternatives here.
Heap
Heap is an auto-capture analytics tool that records all user interactions without requiring a manual event-tracking setup.
Automatic event capture for all user interactions
Retroactive analysis of historical data
User-friendly interface for non-technical team members
Mixpanel
Mixpanel is a powerful analytics platform focused on user behavior analysis and engagement metrics.
Advanced user segmentation and cohort analysis
Real-time data processing and alerting
Customizable dashboards and reports
Amplitude
Amplitude is a product intelligence platform that helps teams build better products through deep user behavior insights.
Behavioral cohorting for targeted analysis
Predictive analytics for user actions and conversions
Cross-platform tracking for a holistic view of user journeys
Conclusion
In conclusion, product analytics is important for understanding how users interact with your product, helping you make data-driven decisions that enhance UX, increase retention, and increase customer satisfaction.
If you are using any of the product analytics solutions mentioned above, check out Iterate AI, an AI-driven event instrumentation platform that only requires PMs to define events and mark where those events should be triggered. Iterate then writes the tracking code snippets for tools like Mixpanel, Amplitude, PostHog, Moengage, etc.; it inserts them into your codebase, and automatically creates a pull request—eliminating the need for developers.
Happy Tracking.